Stochastic Control and Estimation for Linear Systems with Additive Cauchy Noise: Initial Results
January 27, 2012, Webb 1100
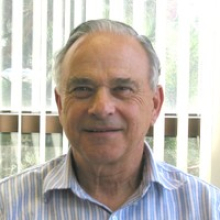
Jason Speyer
UCLA, Mechanical & Aerospace Engineering
Abstract
Uncertainties arising in many practical systems, such as radar glint, have an impulsive character and are better described by non-Gaussian densities with heavy tails. One such heavy tailed distribution is the Cauchy probability density function, which has an undefined mean and an infinite second moment. However, the conditional density function of a Cauchy random variable given a scalar linear measurement with additive Cauchy noise does have a well defined conditional mean and a finite conditional variance, both being functions of the measurement. This is generalized to a scalar linear system where a Cauchy estimator processes an arbitrary sequence of measurements in the presence of Cauchy measurement and process noise. The filter performance is compared with the Kalman filter when the simulation has either Cauchy or Gaussian noise. Next, an optimal multi-step model predictive controller was formulated for scalar discrete-time linear systems with additive Cauchy process and measurement noises, and the solution was compared to an equivalent controller formulated assuming Gaussian noises. The results show that when Cauchy noises are generated for both controllers, the Cauchy controller handles outliers very effectively, whereas the Gaussian controller does not. Interestingly, when Gaussian noises are generated for both controllers, the performance is almost identical. Finally, the conditional mean estimator algorithm is generalized to a two state linear dynamic system with a linear scalar measurement corrupted by additive Cauchy noise. The essential innovations required to generalize the scalar case to the vector case will be described and include the use of the characteristic function of the un-normalized conditional probability density function, an integral formula for exponential function whose argument is the sum of absolute values, and a basis set that produces new elements for the set with each measurement update.
Speaker's Bio
Jason Speyer is a Professor of Mechanical and Aerospace Engineering with a joint appointment in Electrical Engineering. He received his B.S. degree in (Aeronautics & Astronautics from MIT in 1960 and his M.S. and Ph.D. degrees from Harvard University in 1965 and 1968, respectively. He was Harry H. Power Professor of Engineering at the University of Texas, Austin (1976-1989) before moving to UCLA. He is a member of the National Academy of Engineering.
Video URL: