The assignment game: Decentralized dynamics, rate of convergence and the value of information
February 16, 2018, Webb 1100
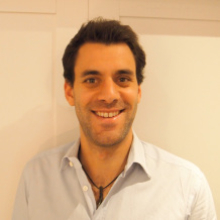
Bary Pradelski
ETH Zurich, Computational Social Science
Abstract
We study decentralized learning dynamics for the classic assignment game with transferable utility but without a central clearing house. At random points in time firms and workers match, break up, and re-match in the search for better opportunities. Agents employ uncoupled learning rules, that is, their strategies are not dependent on other agents’ payoffs or the structure of the game. We propose a simple learning process that converges to stable and optimal outcomes (the core). We then show that naïve strategies are inefficient, that is, the rate of convergence to core outcomes grows exponentially in the number of players. We then discuss behaviorally motivated learning rules that achieve efficiency.
Speaker's Bio
Bary Pradelski is a game theorist who is interested in distributed learning, bounded rationality, and stochastic processes. He is currently a postdoctoral researcher at ETH Zurich in the Department for Computational Social Sciences. Bary was educated in Mathematics at the TU Munich, Ecole Polytechnique Paris, and the University of Oxford from where he received his doctorate for the thesis entitled “Distributed Dynamics and Learning in Games”.
Video URL: