Sensor Selection via Randomized Sampling
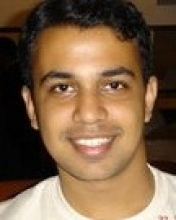
Shaunak D. Bopardikar
Abstract
Speaker's Bio
Shaunak D. Bopardikar is an Assistant Professor with the Electrical and Computer
Engineering Department, and is aliated with the Center for Connected Autonomous Networked
Vehicles for Active Safety (CANVAS) at the Michigan State University. His research interests lie
in scalable computation and optimization, in cyber-physical security and in autonomous motion
planning and control. He received the Bachelor of Technology (B.Tech.) and Master of Technology
(M.Tech.) degrees in Mechanical Engineering from Indian Institute of Technology, Bombay, India,
in 2004, and the Ph.D. degree in Mechanical Engineering from the University of California at
Santa Barbara, USA, in 2010. From 2004 to 2005, he was an Engineer with General Electric India
Technology Center, Bangalore, India. From 2011 to 2018, he was a Sta Research Scientist with
the Controls group of United Technologies Research Center (UTRC) at East Hartford, CT, USA
and at Berkeley, CA. Prior to joining UTRC, Dr. Bopardikar worked as a post-doctoral associate
at UC Santa Barbara (2010-2011) during which he developed randomized algorithms for solving
large matrix games. He is a member of the IEEE Control Systems Society, has over 40 refereed
journal and conference publications and has 2 inventions led for a U.S. patent.